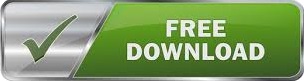
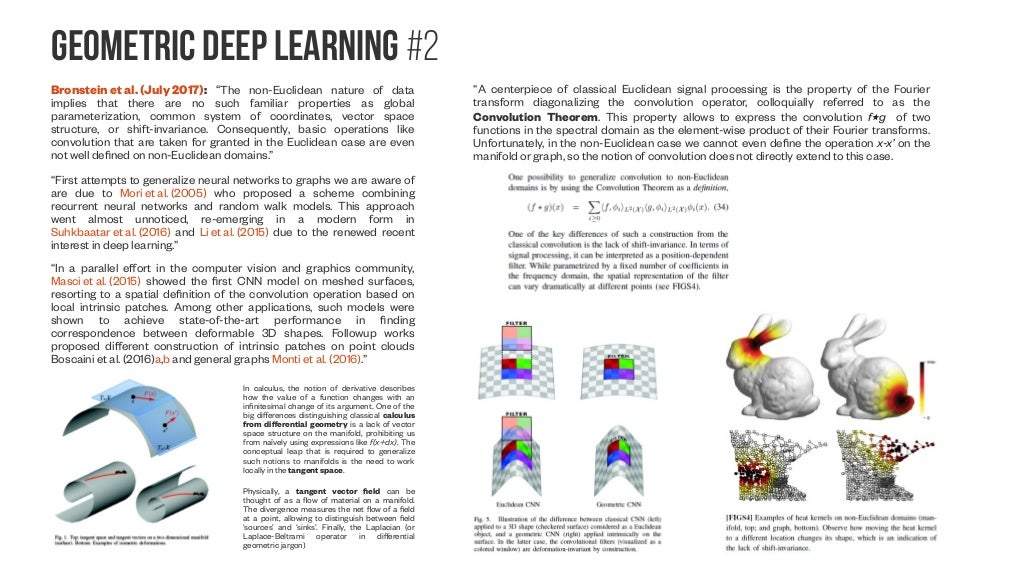
If I move to the left, my location moves to the left. (Or: translation-invariant.) But say the property is location. If I move a few steps to the left, I’m still myself: The essence of being “myself” is shift- invariant. Say the property is some “essence,” or identity - what object something is. The appropriate meaning of “unchanged” depends on what sort of property we’re talking about. SymmetryĪ symmetry, in physics and mathematics, is a transformation that leaves some property of an object unchanged. In the GDL framework, two all-important geometric priors are symmetry and scale separation. Or graphs: The domain consists of collections of nodes and edges. A generic prior could come about in different ways a geometric prior, as defined by the GDL group, arises, originally, from the underlying domain of the task. Geometric priorsĪ prior, in the context of machine learning, is a constraint imposed on the learning task. īefore we get started though, let me mention the primary source for this text: Geometric Deep Learning: Grids, Groups, Graphs, Geodesics, and Gauges ( Bronstein et al. The goal of this post is to provide a high-level introduction. Finally, the rest of us, as well: Even understood at a purely conceptual level, the framework offers an exciting, inspiring view on DL architectures that – I think – is worth getting to know about as an end in itself. Secondly, everyone interested in the mathematical constructions themselves - this probably goes without saying.
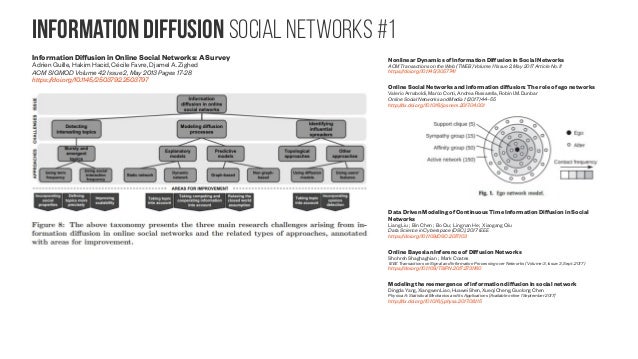
Who, then, should be interested in this? Researchers, for sure to them, the framework may well prove highly inspirational. Prima facie, this is a scientific endeavor: They take existing architectures and practices and show where these fit into the “DL blueprint.” DL research being all but confined to the ivory tower, though, it’s fair to assume that this is not all: From those mathematical foundations, it should be possible to derive new architectures, new techniques to fit a given task. Geometric deep learning (henceforth: GDL) is what a group of researchers, including Michael Bronstein, Joan Bruna, Taco Cohen, and Petar Velicković, call their attempt to build a framework that places deep learning (DL) on a solid mathematical basis. Geometric deep learning: An attempt at unification As for the “elucidate,” this characterization is meant to lead on to the topic of this post: the program of geometric deep learning. Interesting examples exist in several sciences, and I certainly hope to be able to showcase a few of these, on this blog at a later time. By “complement or replace,” I’m alluding to attempts to incorporate domain-specific knowledge into the training process. In this situation, one may feel grateful for approaches that aim to elucidate, complement, or replace some of the magic. Moreover, level of generality often is low. But theory and practice are strangely dissociated: If a technique does turn out to be helpful in practice, doubts may still arise to whether that is, in fact, due to the purported mechanism. Sure, papers abound that strive to mathematically prove why, for specific solutions, in specific contexts, this or that technique will yield better results. Magic, sometimes, in that it even works (or not). More fundamentally yet, magic in the impacts of architectural decisions. Magic in how hyper-parameter choices affect performance, for example. To the practitioner, it may often seem that with deep learning, there is a lot of magic involved. Geometric deep learning: An attempt at unification.LRI is grounded by the information bottleneck principle, and thus LRI-induced models are also more robust to distribution shifts between training and test scenarios. Compared with previous post-hoc interpretation methods, the points detected by LRI align much better and stabler with the ground-truth patterns that have actual scientific meanings. We also propose four datasets from real scientific applications that cover the domains of high-energy physics and biochemistry to evaluate the LRI mechanism. LRI-induced models, once trained, can detect the points in the point cloud data that carry information indicative of the prediction label. This work proposes a general mechanism, learnable randomness injection (LRI), which allows building inherently interpretable models based on general GDL backbones. However, GDL models are often complicated and hardly interpretable, which poses concerns to scientists who are to deploy these models in scientific analysis and experiments. Recently, geometric deep learning (GDL) has been widely applied to solve prediction tasks with such data. Abstract: Point cloud data is ubiquitous in scientific fields.
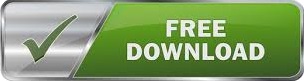